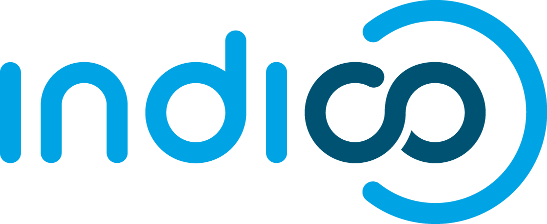
Please log in to access this page.
If you do not have an Indico account yet, you can create one here.
If you do not have an Indico account yet, you can create one here.